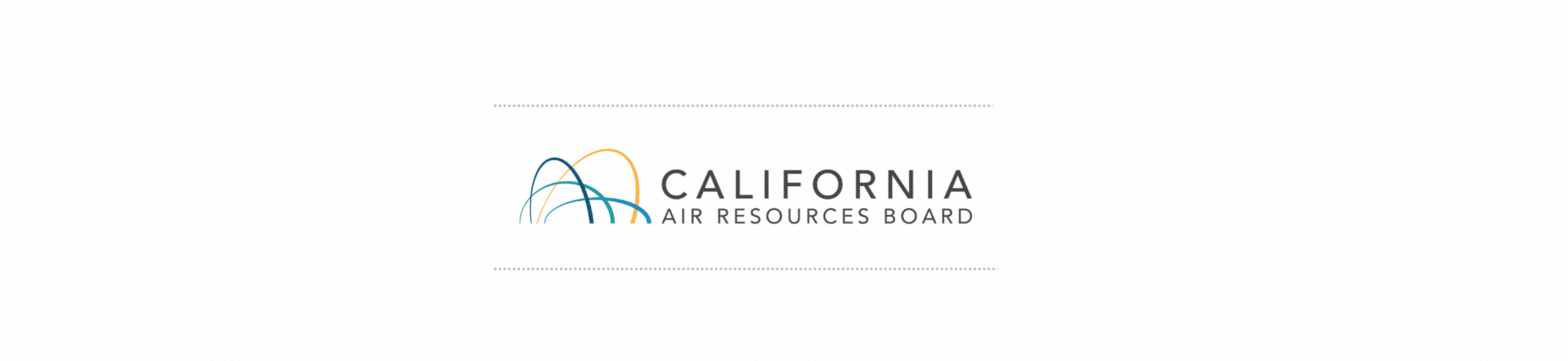
Program Topics:
Our Technical Program Committee has developed several program topics for the 2024 ASIC California Conference that they are excited to build. While the TPC is currently deciding initial speaker invitations, we will be opening the opportunity to give a podium or poster presentation to all the researchers, regulators, communicators, industry members and community scientists that are working on improving air quality sensors.
- Advancing Wildfire Monitoring with Air Quality Sensors — How Air Sensor Data Are Being Integrated at the Local and National Scale
- Air Aware Schools: Protecting Students and Enhancing Learning with Outdoor Air Quality Monitoring
- Air sensor network design and evaluation through an equity lens
- Air Sensors in South Asia — Advances and Updates
- Clean Air Monitoring and Solutions Network: getting useful, actionable data out of low cost sensors for air quality action (CAMS-Net)
- Challenges and successes in community air quality monitoring: implementing and evaluating community-based air quality monitoring programs
- Community-Centric Data Collection: Unleashing the Power of Mobile Sensors
- Community Ownership and Use of Sensor Data
- Effectively converting air quality data to actionable air quality information: Data science tools to scale QA/QC
- Indoor air: Sensors Driving Indoor Air Optimization
- Leveraging low-cost air quality sensors for exposure assessment and health effects research
- Looking beyond London: How Breathe Cities Will Leverage Low-Cost Sensors for Air Quality Management Globally
- Next Generation Air Quality Monitoring Networks
- Next Generation Sensing
- Open Data Workshop Part 1: How data standards enable FAIR and Facilitate exchange of Air Quality Data for Increased Stakeholder Engagement
- Open Data Workshop Part 2: Data Interoperability, Data Exchange, and Data Management
- Sensing and Exposure Assessment of Hazardous Air Pollutants
- Sensors as a component of urban air quality management planning
- The potential of low-cost sensors in regulatory applications: how far have we gone
- Towards a traceable quality of sensor data: from performance standards and test protocols to on-going data quality maintenance procedures
- Weight of Evidence: Building the case for change by combining sensor data with other evidence bases (including e.g. socio-economic data)
The potential of low-cost sensors in regulatory applications: how far have we gone
Low-cost sensors (LCS) have enabled characterization of air quality through measurement of air pollution concentrations at unprecedented scales, underscoring their potentials to reshape air pollution regulations for designing and implementing precise and targeted pollution control strategies. Yet, the well-known performance limitations of low-cost sensors have greatly hindered their use in regulatory air monitoring that requires rigorous data quality standards. This session welcomes submissions that explore innovative, groundbreaking or even transformative approaches to incorporating data from less precise low-cost sensors into regulatory frameworks. All aspects of related sensor applications are invited, which include but are not limited to: novel sensor/monitor design, new calibration approach, innovative data fusion/assimilation method, air pollution policy design based at least partially on low-cost sensor data, among others. Discussion of limitations and gaps in LCS performance that prevent their current use in regulatory monitoring is also encouraged.
Session Chairs:
Raiford Hann, California Air Resources Board, Alastair Lewis, National Centre for Atmospheric Science & University of York, Haofei Yu, University of Central Florida
Podium Presenters:
- Karoline Barkjohn, Physical Scientist, US EPA, United States Presentation PDF
- Helena Pliszka, Air Quality Data Scientist, TD Environmental Services, United States Presentation PDF
- Ron Evans, Senior Analyst, US Environmental Protection Agency, United States Presentation PDF
- Tim Dye, President, TD Enviro, United States Presentation PDF
- Wandji Danube Ngongang, Research Fellow, Kenya
- Michael Hannigan, University of Colorado Boulder Presentation PDF
- Zhi Ning, Hong Kong University of Science and Technology
Presentation titles and abstracts for each of these speakers can be found on the Conference Schedule page.
Community-Centric Data Collection: Unleashing the Power of Mobile Sensors
In an era marked by growing concerns about air quality and its impact on public health, the integration of mobile air sensors into community applications has emerged as a promising tool. This session aims to explore how mobile air sensors are revolutionizing the way communities engage with and address air quality issues. For this session, we seek to bring together experts, researchers, and community leaders who are at the forefront of leveraging mobile air sensors for community-driven air quality monitoring, to explore how mobile air sensors empower communities with real-time air quality data, foster environmental awareness, and drive informed decision-making.
Session Chairs:
Olivia Ryder, Sonoma Technology, Marwa El Sayed, Embry Riddle Aeronautical University, Melissa Lunden, Aclima
Podium Presenters:
- Abdou Safari Kagabo, Assistant Lecturer and PhD Student, University of Rwanda, Rwanda Presentation PDF
- Amirhossein Hassani, Nilu, Norway Presentation PDF
- Dheeraj Alshetty, Advisor Air Quality Scientist, Environmental Defense Fund, India
- Mayra Chavez, Visiting Professor, University of Texas at El Paso, United States Presentation PDF
- Olivia Sweeney, Senior Specialist - Data, Clean Air Fund, United Kingdom Presentation PDF
- Priyanka deSouza, Assistant Professor, University of Colorado Denver, United States
- Janice Lam Snyder, Program Manager, Sacramento Metropolitan Air Quality Management District, United States Presentation PDF
- Thor-Bjørn Ottosen, Danish Technological Institute
Presentation titles and abstracts for each of these speakers can be found on the Conference Schedule page.
Community Ownership and Use of Sensor Data
For air quality sensors to fulfill the promise of helping to improve public and environmental health, data must be effectively communicated appropriately and clearly for a given audience. This means knowing and understanding the audience first and foremost. Effective communication enables data to be used in personal and decision-making, which could inform local actions and policies to improve air quality. To explore this topic, we welcome presentations and posters discussing, but not limited to, the following:
- Public and community partners who have worked with researchers using sensor data – their experience and learnings from case study projects;
- Translating technical details (e.g., regarding air quality science or sensor data quality) into information for public audiences and key lessons learnt;
- Sensor data visualization and best practices.
Session Chairs:
Ashley Collier-Oxandale, Colorado Department of Public Health, Daisha Wall, CleanAIRE NC, Sebastian Diez, Universidad del Desarrollo
Podium Presenters:
- Christi Chester Schroeder, Air Quality Science Manager, IQAir, United States Presentation PDF
- Jackline Lidubwi, Program Coordinator, Internews Network, Kenya
- Kofi Amegah, Associate Professor, University of Cape Coast, Ghana
- Madelyn Percy, Project Coordinator, Education & Community Opportunities Unit, Colorado Department of Public Health & Environment, United States
- Nancy Fitzgerald, Denver Department of Public Health and Environment, United States Presentation PDF
- Savannah D'Evelyn, Postdoctoral Scholar, University of Washington, United States
- Zoe Chafe, C40 Cities Presentation PDF
- Jonathan Callahan, Associate Research Professor, Desert Research Institute, United States
- Gwendylon Smith, Community Health Aligning Revitalization Resilience & Sustainability
- Tajanae Harris, University of Colorado
- Leslie Vasquez, South Bronx Unite
Presentation titles and abstracts for each of these speakers can be found on the Conference Schedule page.
Challenges and successes in community air quality monitoring: implementing and evaluating community-based air quality monitoring programs
Community scale monitoring programs have been rolled out in dozens of locations over recent years because of advances in air sensor technology. New sensor technologies have the potential provide insight into air pollutant concentrations and disparities on neighborhood scales, ultimately yielding valuable information to improve public health. Understanding past challenges and successes is key to building the most effective community monitoring programs in the future. In this session we will explore existing community air monitoring systems with a focus on (1) how new technologies and methods are being applied to tackle monitoring objectives, (2) how programs have complemented and expanded on regulatory monitoring (3) how data has been leveraged to create action, and (4) how program success has been evaluated quantitatively.
Session Chairs:
David Ridley, CARB, Amanda Kaufman, US EPA, William Porter, UC Riverside
Podium Presenters:
- Amirhossein Hassani, Nilu, Norway Presentation PDF
- Caroline Frischmon, Graduate Student, University of Colorado Boulder, United States Presentation PDF
- Ethan McMahon, President, EM Environmental Solutions, United States Presentation PDF
- Evonne Tang, Senior Biological Scientist, US Government Accountability Office, United States Presentation PDF
- Ian Longley, Principal Scientist, NIWA, New Zealand
- John Downie, Technical Business Development Manager, Environmental Instrument, United Kingdom Presentation PDF
- Mohammed Mujtaba, Senior Research Fellow, Kintampo Health Research Centre, Ghana
- Randy Lam, Air Quality Specialist, South Coast AQMD, United States Presentation PDF
- Trinity Vang, Policy and Community Organizer, Brightline Defense, United States Presentation PDF
- Boris Lukanov, PSE Richmond
- Scott Hersey, Associate Professor and Air Partners Lead, Air Partners & Olin College, United States
- Edmund Seto, Professor, University of Washington, United States Presentation PDF
Presentation titles and abstracts for each of these speakers can be found on the Conference Schedule page.
Effectively converting air quality data to actionable air quality information: Data science tools to scale QA/QC
One of the most challenging aspects of running an air quality sensor network is consistently converting real-time, highly spatially-resolved data from a distributed network to information. Transparent data handling processes that scale in support of local air quality monitoring infrastructure are critical to improving the adoption and use of air quality information. In this session we welcome abstracts that explore the development of (1) data science tools around QA/QC for sensor networks, (2) data visualization approaches that enhance dissemination of air quality information, (3) continuous QA evaluation techniques exploring changes in sensor performance over time, including bias and (4) data management, calibration, and control strategies.
Session Chairs:
Alena Bartonova, NILU Climate and Environment Research Institute, Eben Cross, QuantAQ, IQ Mead, Imperial College
Podium Presenters:
- Karoline Barkjohn, Physical Scientist, US Environmental Protection Agency, United States Presentation PDF
- George Castelar, Head of the Air Quality Department, Metropolitan Municipality of Lima, Peru
- Sebastian Diez, Researcher, Universidad del Desarrollo, Chile
- Raiford Hann, Air Resources Engineer, California Air Resources Board, United States
- Amirhossein Hassani, Senior Scientist, NILU, Norway Presentation PDF
- Stig Hellebust, Lecturer, University College Cork, Ireland
- Collins Gameli Hodoli, Postdoctoral Research Associate, University of Georgia, United States
- Arón Jazcilevich, Researcher, Universidad Nacional Autónoma de México, México
- Sebastian Meledina, Environmental Specialist I, New Jersey Department of Environmental Protection Bureau of Air Monitoring, United States Presentation PDF
- Amy Mueller, Associate Professor, Northeastern University, United States Presentation PDF
- Kayla Schulte, Environmental Research Group
- Leonardo Vazquez, Ph.D. Research Associate, The University of Texas at El Paso, United States Presentation PDF
Presentation titles and abstracts for each of these speakers can be found on the Conference Schedule page.
Towards a traceable quality of sensor data; from performance standards and test protocols to on-going data quality maintenance procedures
Despite the common use of AQ sensors, the wider application of sensor data is hampered due to lack of (comparable) evaluation data and due to unknown or questionable performance . Performance standards and validation protocols for different pollutants are being developed to understand sensor performance under controlled and reproduceable conditions. In addition, there is a need to continuously assess sensor performance during their deployment under variable conditions and over time. Therefore, validated and traceable data quality procedures as well as maintenance and calibration protocols are needed.
This session will highlight efforts to develop, compare, and validate performance targets and testing protocols on sensor systems, and on-going data quality control and maintenance procedures. It will address scientific insights on performance parameters, and on how to deal with sensor performance challenges over time. New insights in performance evaluation and on-going data quality procedures for air quality sensors are welcome!
Session Chairs:
Martine Van Poppel, VITO, Edurne Ibarrola, KUNAK
Podium Presenters:
- Brian Stacey, Knowledge Leader, Air Quality Measurements, Ricardo, United Kingdom Presentation PDF
- Gulshan Kumar, Senior Research Scholar, Indian Institute of Technology, India Presentation PDF
- Menaka Kumar, Data Analyst, National Student Services Contract, hosted by the U.S. Environmental Protection Agency, Office of Research and Development, United States Presentation PDF
- Andrea Clements, Physical Scientist, US EPA, United States
- Valerio Ferracci, Senior Scientist, National Physical Laboratory, United Kingdom
- Geoff Henshaw, Aeroqual
- Irene Lara, Kunak Technologies Presentation PDF
- Martine Van Poppel, VITO
Presentation titles and abstracts for each of these speakers can be found on the Conference Schedule page.
Leveraging low-cost air quality sensors for exposure assessment and health effects research
Low-cost air quality sensors provide an accessible and affordable way to assess air pollution exposures, which have implications for health outcomes. Sensors provide opportunities for high spatiotemporal resolution measurement. However, they also present data quality, maintenance, and interpretation challenges. Speakers in this session will share experience and discuss the use of sensors in exposure assessment and health research, including strategies for use, benefits, and challenges.
Session Chairs:
Kofi Amegah, University of Cape Coast, Yisi Liu, University of Kentucky, Liam O'Fallon, NIEHS
Podium Presenters:
- Evan Coffey, Sr. PRA, University of Colorado Boulder, United States Presentation PDF
- Jeff Wagner, Chief, Environmental Health Laboratory Branch, California Department of Public Health, United States Presentation PDF
- Miriam Chacón Mateos, Research Scientist, University of Stuttgart, Germany Presentation PDF
- Naveen Puttaswamy, Assistant Professor, Sri Ramachandra Institute of Higher Education and Research, India
- Tianjun Lu, Assistant Professor, University of Kentucky; California State University, Dominguez Hills, United States Presentation PDF
- Jose Guillermo Cedeño Laurent, Rutgers University
- Sigride Asseko, Oregon State University
- Eric Coker, British Columbia Centre for Disease Control
Presentation titles and abstracts for each of these speakers can be found on the Conference Schedule page.
Indoor air: Sensors Driving Indoor Air Optimization
The COVID-19 pandemic and the ongoing events of smoke from forest fires have brought to fore concerns around indoor air and the role of ventilation, filtration, humidity control, etc in addressing it. The pandemic raised questions around the adequacy of existing guidelines to contain infections indoors and the need for increased outdoor air fraction in the supply line. With the forest fires, however, concerns shifted to limiting outdoor air and filtration. These competing requirements, coupled with demands to improve energy efficiency in buildings, create an opportunity for novel sensing based solutions for control of ventilation systems. In this track, we invite presentations focused on the role of sensors in indoor air monitoring and control.
Session Chairs:
Suresh Dhaniyala, Clarkson University, Randy Chapman, US EPA
Podium Presenters:
- Mst Rowshon Afroz, PhD Student, Environmental and Atmospheric Chemistry, Department of Chemistry, University of Alberta, Canada Presentation PDF
- Chethani Athukorala, Graduate Student, Clarkson University, United States Presentation PDF
- Aynul Bari, Assistant Professor, University at Albany, State University of New York, United States
- Dennis Heidner, Rextor Group Presentation PDF
- Katie Kearns, Technical Director, Berkeley Air Monitoring Group, United States Presentation PDF
- Zhiyuan Li, Associate Professor, Sun Yat-sen University, China
- Tristalee Mangin, Research Assistant, University of Utah Chemical Engineering, United States Presentation PDF
- Theresa Pistochini, UC Davis
- Mahesh Senarathna, Research Assistant, Postgraduate Institute of Science, University of Peradeniya, Sri Lanka
- Brett Singer, LBL
- Zhong-Min Wang, Research Scientist Supervisor, Lab Unit Chief, California Department of Public Health, United States
- Ran Zhao, Assistant Professor, University of Alberta, Canada
Presentation titles and abstracts for each of these speakers can be found on the Conference Schedule page.
Next Generation Sensing
Current air quality sensors focus on criteria air pollutants and have well-known limitations. We invite talks on sensors for emerging and non-regulated pollutants, new technology to measure existing pollutants, and other sensor hardware innovations. Talks may also explore what future sensor developments are needed.
Session Chairs:
R. Subramanian, CSTEP, Karoline Barkjohn, US EPA
Podium Presenters:
- Abhishek Anand, PhD Student, Carnegie Mellon University, United States Presentation PDF
- John Wenger, Professor of Chemistry, University College Cork, Ireland Presentation PDF
- Adil Shah, Postdoctoral Research Scientist, Laboratoire des Sciences du Climat et de l'Environnement, France
- Heather McIntyre, Physical Sciences Researcher/Scientist II, CDPHE APCD ATOPS OMM, United States
- Shwetha Sunil Kumar, PhD Student, Carnegie Mellon University, United States Presentation PDF
- Susanne Hering, Senior Research Scientist, Aerosol Dynamics Inc., United States Presentation PDF
- Xiaobi (Michelle) Kuang, Air Quality Specialist, South Coast AQMD, United States Presentation PDF
- Zeyu Li, PhD Candidate, University of Michigan, China Presentation PDF
Presentation titles and abstracts for each of these speakers can be found on the Conference Schedule page.
Air sensor network design and evaluation through an equity lens
Sensor network design decisions, particularly around sensor siting, are influenced by the objectives of the sensor network. In this session, we will discuss sensor network design when the outcome or focus is on better understanding equity/inclusivity indicators while ensuring effective sensor distribution. We welcome abstracts that discuss the motivation and logistics of sensor siting in this context, approaches for identifying equity/inclusivity indicators that both inform sensor network design and that can be assessed using low-cost sensor data, and results from sensor network deployments.
Session Chairs:
Naomi Zimmerman, University of British Columbia, Yanju Chen, CARB, Dr. Tianjun Lu, California State University Dominguez Hills
Podium Presenters:
- Manish Desai, Research Fellow, Department of Policy & Ethics, School of Medicine, University of Pennsylvania, Philadelphia, PA, USA, United States Presentation PDF
- Edmund Seto, Professor, University of Washington, United States Presentation PDF
- Alex Cabral, Microsoft Research Presentation PDF
- Annemarie Flore
Presentation titles and abstracts for each of these speakers can be found on the Conference Schedule page.
Sensors as a component of urban air quality management planning
How to combine data from regulatory instruments and low-cost sensors for developing and implementing air quality management plans; Local vs regional air quality infrastructure and exploring how these two paradigms of ‘sensing’ pollution present different challenges & opportunities.
Session Chairs:
Lekan Popoola, University of Cambridge, Jin Xu, CARB, Don Collins, University of California Riverside
Podium Presenters:
- Hang Liu, Staff Air Pollution Specialist, CARB
- Imran Shahid, Research Associate Professor, Qatar University, Qatar Presentation PDF
- Miguel Escribano, Business Development Director, BETTAIR CITIES & DNOTA, Spain Presentation PDF
- Scott Epstein, South Coast Air Quality Management District Presentation PDF
- Nuria Castell, Senior Scientist, NILU, Norway Presentation PDF
- Swagata Dey, Program Project Manager, Environmental Defense Fund, India
- Isaac Lino, Air Resources Engineer, California Air Resources Board, United States Presentation PDF
- Ya Wang, Hong Kong University of Science and Technology
- Ian Longley, Principal Scientist
Presentation titles and abstracts for each of these speakers can be found on the Conference Schedule page.
Weight of Evidence: Building the case for change by combining sensor data with other evidence bases
How to combine data from regulatory instruments and low-cost sensors for developing and implementing air quality management plans; Local vs regional air quality infrastructure and exploring how these two paradigms of ‘sensing’ pollution present different challenges & opportunities.
Session Chairs:
Ethan McMahon, EM Environmental Services, Rebecca Garland, University of Pretoria and ANGA, Albert Presto, Carnegie Mellon University
Podium Presenters:
- Douglas Booker, Co-Founder & CEO, NAQTS / Lancaster University, United Kingdom Presentation PDF
- Karen Grzywinksi, ACCAN
- Amirhosein Mousavi, Research Associate, University of Southern California, United States Presentation PDF
- Daniel Westervelt, Associate Research Professor, Columbia University, United States Presentation PDF
Presentation titles and abstracts for each of these speakers can be found on the Conference Schedule page.
Next Generation Air Quality Monitoring Networks
The current paradigm for using low-cost sensors typically involves calibrating and deploying one or more sensors, collecting data, and analyzing these data in isolation to determine air quality at each sensors’ location. This session will feature presentations about how we might move beyond that, and what the next generation of air quality monitoring networks might look like. We encourage presentations about innovative approaches which combine data from multiple sensors across a network, and potentially also external systems such as satellite remote sensing instruments or atmospheric transport and chemistry model outputs, to best use the strengths of these different approaches to build up our overall understanding of air quality. Potential topics include data assimilation or data fusion with low-cost sensor data, air quality forecasting with low-cost sensor networks and meteorological data, and strategies for in-situ performance verification or re-calibration of low-cost sensors using network-level data quality metrics. Note that this session is not designed for presentations on new sensor technologies (these should be submitted to the “future sensor development needs” session) nor for presentations about the use of sensors together with socioeconomic data (these should be submitted to the “weight of evidence” session).
Session Chairs:
Carl Malings, NASA, Haofei Yu, University of Central Florida
Podium Presenters:
- Ben Crawford, Assistant Professor, University of Colorado Denver, United States
- Daniel King, Geospatial Data Scientist, Sonoma Technology, Inc., United States
- Daniel Westervelt, Associate Research Professor, Columbia University, Lamont Doherty Earth Observatory, United States Presentation PDF
- Haris Sefo, Head of Research and Sensor Systems Development, Breeze Technologies UG & HCU University Hamburg, Germany Presentation PDF
- Jiayu Li, Universty of Miami Presentation PDF
- Ajit Ahlawat, Research Scientist, Leibniz institute for tropospheric research, Germany Presentation PDF
- Lovanomenjanahary Marline, Post doctoral fellow, Association Vahatra, Madagascar
Presentation titles and abstracts for each of these speakers can be found on the Conference Schedule page.
Sensing and Exposure Assessment of Hazardous Air Pollutants
The advent and widespread use of low-cost sensors have dramatically improved the ability of communities to measure their exposure to criteria air pollutants such as particulate matter, ozone, and nitrogen oxides. However, many underserved communities also face exposure to hazardous air pollutants (HAPs), such as toxic metals and volatile organic compounds (VOCs). This session aims to bring together researchers, community members, industrial partners, and government agencies to discuss the latest advancements and challenges in ambient monitoring of and exposure assessment to HAPs. The session will cover a wide range of topics including, but not limited to: 1) Technological advancements that enable near real-time monitoring of toxic metals and VOCs; 2) Identification of HAPs trends and source apportionment; 3) Use of remote sensing technologies to achieve monitoring of HAPs at high temporal- and spatial resolution; 4) Use of ambient HAPs measurements for exposure assessments and air quality model evaluation.
Session Chairs:
Hanyang Li, San Diego State University, Serena Chung, US EPA
Podium Presentaters:
- Ashley Collier-Oxandale, ATOPS Data & QA Unit Supervisor, Colorado Department of Public Health & Environment APCD, United States
- Caroline Frischmon, Graduate Student, University of Colorado Boulder, United States Presentation PDF
- Detlev Helmig, Principal, Boulder AIR, United States
- Emilio Molina Rueda, Research Assistant, Colorado State University, United States Presentation PDF
- Wilton Mui, AQ-SPEC Program Supervisor, South Coast Air Quality Management District, United States Presentation PDF
- Zheyuan Pei, Graduate Research Assistant, University of Utah, United States Presentation PDF
- Wayne Linklater, Professor and Chair, Dept. of Environmental Studies, California State University - Sacramento, United States Presentation PDF
- Megan MacDonald, US EPA, Office of Research and Development
Presentation titles and abstracts for each of these speakers can be found on the Conference Schedule page.
Clean Air Monitoring and Solutions Network: getting useful, actionable data out of low cost sensors for air quality action
The Clean Air Monitoring and Solutions Network (CAMS-Net) establishes an international network of networks that unites scientists, decision-makers, city administrators, citizen groups, the private sector, and other local stakeholders in co-developing new methods and best practices for real-time air quality data collection, data sharing, and solutions for air quality improvements. CAMS-Net brings together a vast network of multidisciplinary member networks from all around the globe. The project establishes a mechanism for international collaboration, builds technical capacity, shares knowledge, and trains the next generation of air quality practitioners and advocates, including domestic and international graduate students and postdoctoral researchers. We welcome presentations on topics including but not limited to:
- calibration/correction factor development
- data sharing, management, and standardization
- application of sensor data to modeling and satellite data
- role of sensors in source attribution
- public engagement and capacity building
- decolonization of science and responsible science
- public policy and health
Session Chairs:
Dan Westervelt, Columbia University, Albert Presto, Carnegie Mellon University
Podium Presentaters:
- Abid Omar, Founder, Pakistan Air Quality Initiative, Founder, Pakistan
- Collins Gameli Hodoli, Postdoctoral Research Associate, University of Georgia, Athens, United States
- Garima Raheja, PhD Candidate, Columbia University, United States
- Daniel King, Sonoma Technologies, United States
Presentation titles and abstracts for each of these speakers can be found on the Conference Schedule page.
Air Aware Schools: Protecting Students and Enhancing Learning with Outdoor Air Quality Monitoring
This panel discussion will shed light on the importance of monitoring outdoor air quality at educational institutions and share lessons learned from a wide variety of school-related air quality monitoring efforts. Join us as our panelists explore the transformative role of real-time air quality data in safeguarding the well-being of students and staff, enhancing the overall learning environment, and engaging the broader community in air quality advocacy.
The session will begin with brief presentations from each panelist on their areas of focus, followed by a panel discussion with questions from the audience for the entire panel.
- Bill Obermann will share how the Love My Air program’s mission to provide accessible and actionable real-time air quality information is being used by school nurses and teachers in Denver Public Schools. Highlights will include a summary of our ‘teach the teacher’ model, partnering with local environmental justice-focused nonprofits to provide before and after school education, and the next generation of Love My Air monitoring and communication technologies.
- Sarah Kroening will discuss the Children's Health Alliance of Wisconsin’s partnership with the City of Denver to replicate the Love My Air Denver program in Wisconsin. She will share information about the EPA grant award to create a community-based network of neighborhood-level air quality monitoring stations by partnering with Milwaukee Public Schools. She will share the challenges and successes of the program’s first year. Additionally, Sarah will explain the use of air sensor data to gain knowledge and build awareness around localized air quality data, partnerships with local and state organizations, and the vision to develop Love My Air Wisconsin for schools across the state.
- Carey Jensen from Boulder Valley School District, who after the devastating Marshall Wildfire ripped through their community, partnered with Boulder County Public Health to quickly deploy air quality devices at all 8 schools in the fire area. As the District continues to recover, these monitors are equipped with live updates and alerts.
- Story Schwantes will discuss how air quality is being integrated as part of the educational curriculum through the AQEarth program in Fort Collins and Anchorage, Alaska and the Youth Air Quality Advocates program in San Jose, California.
- Dr. Joe Lyou, President & CEO at Coalition for Clean Air will share the work they have done to advocate for air quality awareness at schools across California, as well as their role in building the largest school-based air quality monitoring network in the country at LAUSD.
- Carlos Torres from Los Angeles Unified School District, who deployed the "Know Your Air" network, a collaborative effort between the Los Angeles Unified School District, the Coalition for Clean Air, and Clarity Movement Company, will discuss how their network of more than 200 air quality sensors provides real-time, local air quality data to protect the health and well-being of Los Angeles Unified's vast community of more than 700K students as well as teachers, administrators, and other employees.
- Join us to learn how these projects are empowering schools to bring air quality into the classroom, make data-informed decisions, and create a healthier and more productive atmosphere for their communitie
Session Chairs:
Ryan Higgins, Clarity & Sarah Kroening, Children's Health Alliance of Wisconsin
Panel Speakers:
- [Moderator] Aubrey Burgess - Projects Team Lead at Clarity Movement
- Sarah Kroening - Program Manager of Love My Air WI with Children's Health Alliance of Wisconsin
- Story Schwantes, Air Quality Science Project Manager at TD Enviro
- Dr. Joe Lyou, President & CEO at Coalition for Clean Air
- Carlos Torres, Director - Office of Environmental Health & Safety at LAUSD
- Bill Obermann - Air Program Supervisor, Department of Public Health and Environment with City and County of Denver
Presentation titles and abstracts for each of these speakers can be found on the Conference Schedule page.
Advancing Wildfire Monitoring with Air Quality Sensors — How Air Sensor Data Are Being Integrated at the Local and National Scale
In this panel discussion, we delve into the use of air quality sensors for wildfire monitoring, with a focus on enhancing public safety and response strategies during wildfire events. Our panel of experts will share their invaluable insights on the deployment of low-cost air quality sensors — at both the local and the national level — to address the distinct challenges posed by wildfires.
Air quality sensors that offer durability, ease of deployment, and real-time data can be invaluable for the development of effective wildfire smoke monitoring and alerting systems. While acknowledging the challenges associated with some low-cost sensors, our discussion will highlight the potential for reliable sensor technology in wildfire monitoring, especially in scenarios where government agencies can lead the way in implementing necessary changes.
We will hear from two agencies —Monterey Bay Air Resources District and Boulder County Public Health — about their experiences leveraging sensors to respond to extreme air pollution events. Steven Brown will also provide an overview of the mobile monitoring for VOCs and benzene performed by NOAA during the Marshall Fire in Boulder — yielding findings regarding wildfire smoke composition beyond just particulate matter.
The panel will also explore the integration of data from low-cost air sensors into the EPA Fire and Smoke Map, a collaborative effort between the U.S. Forest Service (USFS) and the U.S. Environmental Protection Agency (EPA). The map provides crucial information to protect public health from wildfire smoke, and its data sources include AirNow, the Western Regional Climate Center, AirSis, and low-cost air sensors, reinforcing the growing importance of the integration of data from low-cost air quality sensors in wildfire management. Join us for an insightful discussion on the future of wildfire monitoring and public safety through the power of air quality sensors
Session Chairs:
Ryan Higgins, Clarity; Levi Stanton, Clarity; Ron Evans, US EPA
Panel Speakers:
- [Moderator] Levi Stanton, Solutions Engineering Lead at Clarity Movement (levi@clarity.io)
- Ron Evans from the US EPA Office of Air Quality Planning and Standards (evans.ron@epa.gov) Presentation PDF
- Sim Larkin, Climate Scientist and AirFire Team Leader at US Forest Service (sim_larkin@firenet.gov)
- David Frisbey, Planning and Air Monitoring Manager from MBARD (DFrisbey@mbard.org) Presentation PDF
- Matthew Coggon, Research Chemist, Tropospheric Chemistry at the NOAA Chemical Sciences Laboratory (matthew.m.coggon@noaa.gov) Presentation PDF
- Bill Hayes, Air Quality Program Director at Boulder County Public Health (bhayes@bouldercounty.gov) Presentation PDF
Presentation titles and abstracts for each of these speakers can be found on the Conference Schedule page.
Looking beyond London: How Cities Will Leverage Low-Cost Sensors for Air Quality Management Globally
The Breathe London air quality monitoring program — led by the expert team at Imperial College London’s Environmental Research Group — is a success story that offers a flexible and applicable framework for achieving cleaner air in urban areas globally.
In this panel discussion, we'll share lessons learned from deploying more than 450 air quality sensors (and growing) across London — including how to build the awareness and partnerships that breathe life into the program. We’ll examine the critical elements that have led to the program’s sustained success — including the operational mode of the team running the network, funding, technical support, data sharing, community engagement, and capacity building — and share how the Breathe London model offers a blueprint for cities looking to establish robust, high-resolution air quality monitoring programs worldwide
Session Chairs:
Ryan Higgins, Clarity & Iq Mead, Imperial College London
Panel Speakers:
- [Moderator] Sean Wihera, Vice President, Business Development and Partnerships at Clarity Movement Presentation PDF
- Andrew Grieve, Senior Air Quality Analyst at the Environmental Research Group, Head of Air Quality Measurement in the ERG team, Imperial College London
- Dr. Kayla Schulte, Postdoctoral Research Associate, Environmental Research Group, Imperial College London
Presentation titles and abstracts for each of these speakers can be found on the Conference Schedule page.
Air Sensors in South Asia — Advances and Updates
There has been tremendous growth in the use of low(er) cost air quality sensors in low- and middle-income countries, especially in South Asia. Presentations during this session will describe sensor deployments and their intent and highlight both successes and challenges and share data from deployments to date. We expect abstracts focusing on both technical details of deployments and on applied findings from these deployments. This session will both share findings from existing deployments and share learnings with those seeking to deploy these types of networks around the world.
Session Chairs:
Ajay Pillarisetti, UC Berkeley, R. Subramanian, CSTEP, Swagata Dey, EDF
Podium Presenters:
- Kalpana Balakrishnan
- Rashmi Krishnan
- Swagata Dey
- Emil Varghese
- Mark Campmier, SAMOSA
- Asim Jaffry, Fair Finance Pakistan
Presentation titles and abstracts for each of these speakers can be found on the Conference Schedule page.
Open Data Workshop Part 1: How data standards enable FAIR and Facilitate exchange of Air Quality Data for Increased Stakeholder Engagement
The first part of this two-part workshop will focus more on the “why” of open data and the front-end data use. Open data serves as a connective mechanism for collaboration between different sectors of society. Universal and open access to air quality data promotes change and empowers community members to access, understand and advocate for solutions for clean air. While “openness” can be a spectrum, truly open data should meet guidelines that make it findable, accessible, interoperable, and reusable (FAIR). Part 1 will explore the findable, accessible, and reusable aspects of this definition, while Part 2 will delve more deeply into interoperability. Open data instills transparency and accountability; moreover, it fosters collaboration and promotes equity, especially for disproportionately impacted communities to advocate for equitable solutions. For air quality data, what opportunities and barriers exist in making data open? What tools exist for stakeholders—civil society, industries, governments, nonprofit organizations—to address apprehensions and reticence, and enhance open data access? Speakers in Part 1 of this workshop will share concrete examples to facilitate discussion around these questions.
Session Chairs:
Colleen Rosales & Vasu Kilaru, EPA
Workshop Panel:
- Olivia Sweeney, Clean Air Fund Presentation PDF
- Vasu Kilaru, EPA
- Abid Omar, Pakistan Air Quality
Presentation titles and abstracts for each of these speakers can be found on the Conference Schedule page.
Open Data Workshop Part 2: Data Interoperability, Data Exchange, and Data Management
Given the variety of manufacturers, the costs of integrating data from a variety of sources can be substantial both for general users and especially for community-based organizations with limited data science expertise. Data exchange is often hampered by differences in things like file formats, parameter names, file types. The lack of metadata that includes data quality parameters also makes data sharing and harmonization challenging. Additionally, Data Management Systems (DMSs) remain complex, often proprietary, and costly. In the second part of this two part workshop, we will focus on the back-end mechanics of open data and data interoperability. For example, the Colorado Department of Public Health and Environment's APCD, in partnership with the US EPA and TDEnviro, has developed a draft standard that aims to offer a single format to capture key information about data generation and facilitate data exchange - the Air Quality Data Exchange (AQDx) format. As another example, the Clean Air Fund conducted a worldwide assessment of the needs for a DMS’s. Findings illustrated the need to establish a community of practice around this topic, develop open-source software to streamline ingest, quality control, processing, and distribution of air quality data. Other findings included key features (e.g., data aggregation, data security, QC tools, and basic visualization) and the need for data harmonization. This workshop will offer us an opportunity to identify and discuss existing efforts, including the two mentioned here, to solve these challenges, coordinate among these efforts as appropriate, and establish a network for progressing on these issues going forward. To help facilitate the discussion, a survey will be sent out ahead of the workshop to registered ASIC attendees asking about specific tools and projects supporting data interoperability, data exchange, and data management. We are at a unique moment in time with the convergence of the need for open data, funding, and collaborative people and organizations seeking to make data open and accessible for all.