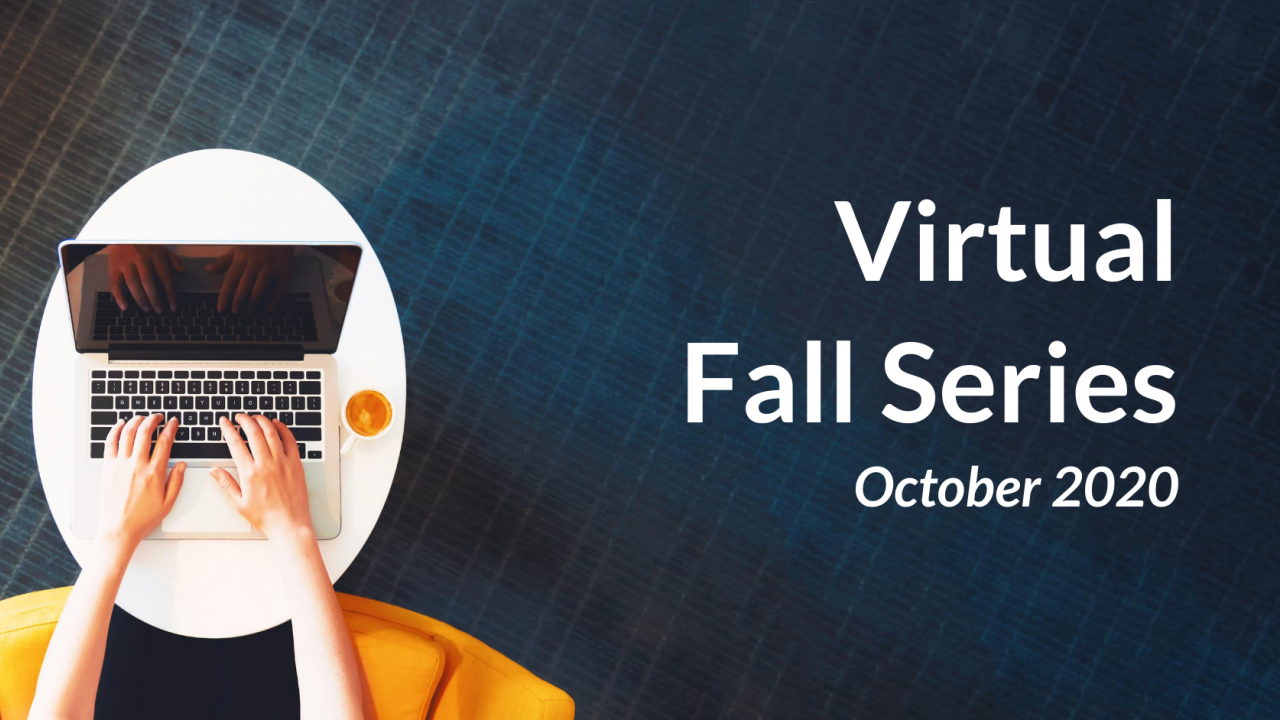
Event Date
Session moderated by Ethan McMahon, US EPA & Ajith Kaduwela, CARB
New Ambient Sample Capture Apparatus Provides Speciation Methods using Next Generation Air Monitoring Networks
Presented by: Ken McGary, Apis-US, Inc.
Summary: Performance of modern low-cost sensor networks is fast approaching the realm of full-on reference instruments. Electrochemical and PID gas detection levels are now often in the low single-digit-ppb domain, and inexpensive optical particle counters can show impressive correlation to reference-grade analyzers. However, a major drawback remains the inability to speciate detected pollution levels of both Volatile Organic Compounds VOCs) and Particulate Matter (PM).
Apis solves this problem with our Data Fusion platform, using sensitive broadband sensors, sophisticated anomaly detection, and our new Capture Box accessory to collect ambient air samples for lab analysis using tried and trusted methods. For VOCs this means triggered subatmospheric pressure sampling with SUMMA canisters and GC/MS back in the lab. For particulates, a triggered hi-volume sampler such as the Tisch Environmental HiVol can be used to collect samples for various physical or chemical characterizations.
In either case, the time-consuming hit-and-miss nature of ambient sampling is refocused on sampling during pollution “events” recognized in real time, vastly increasing the efficacy and cost-effectiveness of these expensive analytical procedures. These selective sampling techniques are already paying dividends in a Colorado sensor network deployment near oil and gas drilling sites. We are now also deploying VOC capture stations in a variety of industrial and community projects, and are looking for new partners to further expand the usefulness of this new paradigm based on well-established analytical methods.
Observing urban CO2 emission changes from the transportation sector: COVID-19 and beyond
Presented by: Alex Turner, UC Berkeley
Summary: We describe application of the Berkeley Environmental Air-quality and CO2 Observation Network (BEACO2N) to quantify trace gas fluxes in an urban area. We quantify decreases CO2 emissions due to mobility restrictions from shelter-in-place orders due to COVID-19. BEACO2N has ~70 nodes on 2km spacing in the SF-Bay Area. Every node in the network observes an enhancement associated with the surrounding region. We couple a weather model with a back trajectory model to determine this region of influence. Following this, we use Bayesian inference to derive emissions before and during shelter-in-place. Additional effects such as the role of vehicle speed will be discussed.
Determining fleet-based vehicle emission factors using low-cost sensors: A case study in three parking garages
Presented by: Naomi Zimmerman, University of British Columbia
Summary: Traditionally, vehicle emissions measurements rely on reference-grade instruments whose high cost and complexity have limited their deployment in real-world environments. New simple-to-operate, low-cost sensing technologies are a potential solution to this problem. To assess their suitability, we deployed six Sensit Real-time, Affordable, Multi-Pollutant (RAMP) monitors measuring PM2.5, NO, NO2, CO2, O3 and CO in three parking garages on the UBC Vancouver campus from April–August 2019. UBC Parking Services provided real-time vehicle counts to help validate our method. After sensor calibration, integrated pollutant and CO2 signals were converted to fuel-based emission factors (EFs). Our calculated EFs fell within the range of previous studies. Evening EFs when vehicles were cold were 10-50% higher than in the morning. We also observed a disproportional contribution of high emitters; the top 25% of plumes contributed 45-65% of total emissions. Our findings indicate that low-cost sensors are a promising technology for real-world vehicle emissions measurement.
Evaluation of Low-Cost Particulate Matter Sensors for Measuring Wildfire Smoke
Presented by: Dena Vallano, US EPA
Summary: Until recently, most wildfire air quality impacts were determined by permanent stationary regulatory monitors that are used to calculate the Air Quality Index (AQI). Low-cost particulate matter (PM) sensors have found widespread use by the public in smoke impacted areas but have not been evaluated at the high smoke concentrations frequently encountered near wildfires. We collocated three low-cost PM/air quality sensor systems (Aeroqual – AQY1, PurpleAir - PAII-SD, Sensevere - RAMP) with reference PM monitors near three wildfires in the western U.S. and one prescribed fire in the eastern U.S. (max PM = 295 µg/m3). The sensors were moderately - highly correlated with the reference monitor (hourly averaged r2 = 0.52-0.95). All sensors overpredicted PM2.5 concentrations, with an average normalized mean bias of 41%, 62%, and 40% for AQY1, PAII-SD, and the RAMP respectively.
Calibration factors for individual fires varied, likely due to the different concentration ranges observed at each fire. By combining all datasets, a smoke specific calibration factor was developed that reduced the normalized root mean square error to less than 35%. The calibration factors varied among the sensors, demonstrating the impact of the physical configuration of the sensor and the algorithm used to translate the size and count information into PM concentrations. These results suggest the low-cost sensors tested here can fill in the large spatial gaps in monitoring networks near wildfires with errors of less than 10 µg/m3 in the hourly PM2.5 concentrations when using a sensor specific smoke calibration factor.
This abstract was reviewed by EPA and approved for publication, it may not necessarily reflect official Agency policy. Mention of trade names or commercial products does not constitute endorsement or recommendation for use.
The Africa qualité de l'Air network (AfriqAir): A continent-spanning air quality monitoring network using lower-cost sensors
Presented by: R. Subramanian, QEERI & OSU-Efluve
Summary: Ambient air pollution is a leading cause of premature mortality with an estimated 258,000 deaths in Africa (UNICEF/GBD 2017). Such estimates have large uncertainties as many major cities in Africa do not have any ground-based air quality monitoring. Without granular data, it is impossible to quantify the magnitude of air pollution and its impacts, determine pollution sources, and develop and evaluate interventions. The lack of data is due in part to the high cost of traditional monitoring equipment and a lack of trained personnel.
We propose filling this data gap with a hybrid network of low-cost sensors combined with (and carefully calibrated to) reference monitors. About 50 real-time affordable multi-pollutant (RAMP), Clarity, and PurpleAir devices have been collaboratively deployed in Nairobi, Kigali, Accra, Abidjan, Niamey, Kinshasa, Zamdela (near Johannesburg), and other cities. All use Plantower optical nephelometers for PM2.5. RAMP and Clarity use Alphasense electrochemical sensors for NO2. Local partnerships will ensure that the sensors are well-maintained and sites determined by local experts. We will also work to develop local capacity to ensure network sustainability.
Using a calibration developed in Creteil, France, the deployments reveal morning and evening spikes in combustion-related air pollution. Median hourly NO2 in Accra and Nairobi for September-October 2019 was ~11 ppb; a similar value was observed in November-December 2019 in Zamdela. However, pilot studies in Rwanda showed that local calibration is crucial. So we acquired regulatory-grade PM2.5, NO2, and O3 monitors for Abidjan, Accra, and Nairobi. We also collocated lower-cost devices with existing reference monitors and/or integrated passive samplers in Zamdela, Kigali, Abidjan, Kampala, and Lamto. We shall present preliminary results on spatio-temporal variability of collocation-based sensor calibrations, source identification, and challenges and plans for future expansion.
Scaling-up Low-cost Sensor Evaluation and Network Integration: the Paris / Ile-de-France Experience
Presented by: Adrian Arfire, Airparif
Summary: Airparif, the observatory in charge of monitoring and informing on air quality in the Parisian region, accompanies the stakeholders and public authorities. Thus, data reliability is essential to settle appropriate action for improving air quality. Over the past years, the technological advancements of pollution sensors enable the development of novel networks that equip buildings, street furniture, vehicles or citizens. Nevertheless, the appropriation of the measurement by the general public does not automatically imply becoming a specialist of air quality. As such, a real risk is emerging of data privatization and disqualification of experts. The multiplication of data sources is also a great opportunity for the evolution of air quality monitoring. Airparif end goal is to be able to channel and integrate the data generated by these diverse measurement sources within a unified monitoring framework, in the service of the collective interest. To this end, appropriate answers to the following questions need to be found: How to evaluate the reliability of these sensors? How to collect and assimilate the data in order to improve accuracy and coverage and to provide reliable local information? What is the specific contribution that these novel technologies can bring to monitoring, communication, and raising awareness? In the absence of norms and performance standards, Airparif has developed, through its platform for open innovation – AIRLAB, an evaluation methodology based on the principle of the challenge, by coupling metrology and ergonomics criteria. Multiple projects involving fixed, mobile, or portable low-cost sensor systems are also currently deployed by Airparif. These field experimentations allow us to draw initial insights into the potential gain obtained through sensor multiplication in terms of monitoring, personal exposure characterization, and education.
Open Source Edge Computing Platform for Air Quality Applications
Presented by: Sai Yamanoor, DesignAbly
Summary: Citizen Science or Community Driven efforts can provide data that can be used to predict or identify common and repeatable events. For e.g.: Images collected from community experiments have been used to identify certain insect species etc. Also, satellite images have been used to predict start of wildfires. Likewise, it is possible to use data community collected data to predict events in Air Quality Monitoring or detect anomalies. Powerful computing resources are required to build and train a model to predict events. With the recent advancements in the field of Machine Learning and Computing power, it is possible to run neural networks on hardware that costs less than $20. This enables conducting analysis on the collected data at the source instead of transferring it to a centralized “data lake.'' It also reduces costs as it helps avoid certain cloud computing and related infrastructure costs. This concept of conducting the data analysis at the source instead of the cloud is called Edge Computing. Edge computing can be a great resource in citizen science experiments because it helps avoid “reinventing the wheel”. In citizen science applications, the arrival of lost cost edge computing hardware enables running community driven experiments at a low cost. This also enables verify or re-emulating results observed in pre-existing datasets. In our presentation/poster, we are proposing an open source edge computing platform for citizen science experiments. The platform is centered around the Artemis module that costs US$8. The platform comes with interfaces to accommodate sensors with different outputs like UART, I2C etc. We would also like to demonstrate a use case for the platform using an existing dataset.