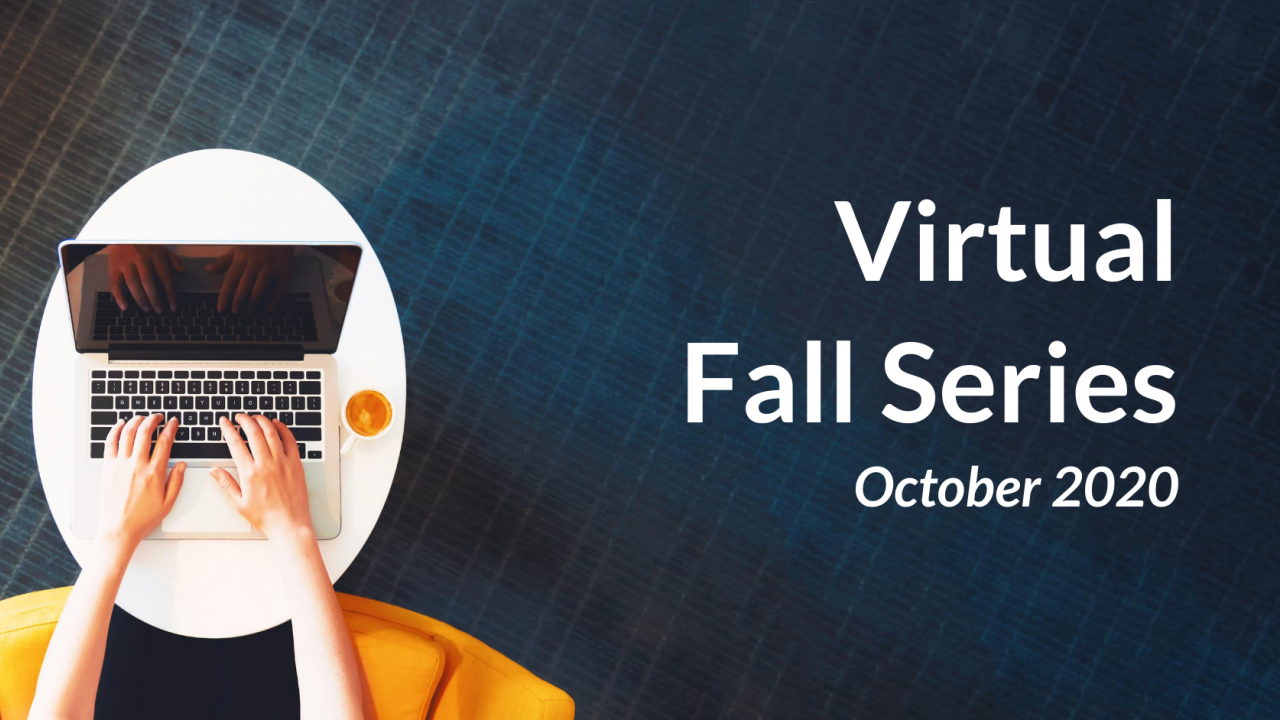
Event Date
Session moderated by Ethan McMahon, US EPA and Tim Dye, TD Environmental
Regulatory Comparison of Low Cost OPC Instrumentation for Ambient Particulate Mass Concentration Measurement
Presented by: Jen Brown, Met One Instruments, Inc.
Summary: This experimental study compares regulatory PM1.0, PM2.5, and PM10 mass concentration measurements with results from real-time optical particle counter (OPC) mass measurement devices. Total mass in the form of PM1.0, PM2.5, and PM10 was continuously measured during the event using US-EPA FEM designated beta attenuation mass monitors “BAM”. Three “BAM” units were collocated with three OPC mass measurement devices at each of three different locations: Elizabeth NJ, Salt Lake City UT, and Riverside CA. Data comparisons include hourly and 24-hour averages. Analysis of data from May 2019-June 2020 reveal excellent PM10 linear correlation coefficients of 0.79, 0.90, and 0.91; PM2.5 linear correlation coefficients of 0.65, 0.77, and 0.75; and PM1.0 linear correlation coefficients of 0.51, 0.72, and 0.64 for each respective site (NJ, UT, CA). OPC versus “BAM” slopes differ between mass size concentrations at each site, as well as when comparing the same mass size cut point between different sites. This provides evidence of the importance of a k factor to accommodate for dissimilar ambient conditions at different sites. Flow is critical to the accuracy of optical particle counters; flow stability is vital to minimize drift. The OPC mass measurement units maintained excellent intra-model precision across the study, and this is attributed to its enhanced internal flow system. Meteorological parameters have been investigated to explain occasional large shifts from FEM reported particulate concentration measurements.
Application of Electrochemical Analysis Methods to Diagnose Atmospheric Impacts on Electrochemical Gas Sensor Response
Presented by: Anna Farquhar, Aeroqual Ltd.
Summary: Amperometric electrochemical gas sensors are widely used for the detection of gases in air quality instruments. Such sensors generate output currents that are proportional to the target and cross-interference gas concentrations under diffusion controlled conditions. The sensor is also influenced by atmospheric conditions particularly temperature and relative humidity. Changes in temperature or humidity can cause both transient and slow drift in the output current. This presentation will discuss the use of electrochemical methods to characterize commercial electrochemical sensors before, during, and after a sudden change in the atmospheric condition. In situ electrochemistry allows the determination of how important sensor characteristics (active surface area, electrolyte resistance, electrode contamination etc.) change with atmospheric conditions in real-time. This work gives a better understanding of how electrochemical sensors react to their environment, and enables the development of diagnostic tools to evaluate sensor health and predict sensor response under changing climatic conditions.
Long-Term Performance of Five Air Sensor Models Across Seven U.S. Sites
Presented by: Karoline Johnson Barkjohn, US EPA
Summary: For any single project, air sensor performance is typically evaluated at a single site or within a single region over a short time period (weeks to months). However, sensor performance often depends on environmental conditions, pollutant concentrations, and particle properties. In addition, performance may change due to seasonal changes in pollutant mixtures or environmental conditions and degradation of the sensor performance. This makes it difficult for potential users to decipher expected performance for their location and conditions. With this in mind, in August 2019 five types of air sensors were set up for a yearlong collocation at seven air monitoring stations across the United States. Sensors were located at stations across climate regions of the U.S. including North Carolina, Georgia, Delaware, Arizona, Colorado, Oklahoma, and Wisconsin. The five sensor models were the Clarity Node from Clarity Movement, PA-II-SD from PurpleAir, AQY1 from Aeroqual, Maxima from Applied Particle Technologies, and the RAMP from SENSIT. The selected sensors measure either particulate matter (PM), or a combination of PM and some gas pollutants. The performance of the PM2.5 and ozone (O3) measurements will be discussed. Sensors were evaluated for accuracy, precision, and the influences of environmental conditions including temperature and relative humidity. Accuracy was evaluated monthly at each site so that comparisons could be made over time and across sites. The results from the first six months of the project provide valuable insights into the performance of air sensors in different climates, including changing seasons. In addition, broader lessons learned about in-field sensor management, data completeness, and data management will be discussed.
Although this abstract was reviewed by EPA and approved for publication, it may not necessarily reflect official Agency policy. Mention of trade names or commercial products does not constitute endorsement or recommendation for use.
A novel calibration method for hyperlocal measurements of air quality using a low-cost sensor network
Presented by: Lekan Popoola, University of Cambridge
Summary: Low-cost sensors are now increasingly being used in air quality research including emission source identification, ambient air pollution monitoring for assessment and human exposure studies. Although the benefits of low-cost sensors are recognised, one of the main challenges has been their ease and accuracy of calibration, particularly when deployed as dense networks. These devices are typically calibrated in the field by either collocation with reference instruments or by using transfer of calibrated standards approach (collocations with pre-calibrated low-cost sensors). While these methods are reliable, they present logistic challenges, often taking a long time to complete. In this paper we present a cloud-based calibration method developed at University of Cambridge as part of the Breathe-London (BL) project.
The Breathe-London project (https://www.breathelondon.org) combines state-of-the-art measurement technology with over 100 low-cost sensor nodes (each measuring NO, NO2, O3, and CO2, PM2.5 and PM10) along with two Google Street View cars instrumented with reference grade air quality instruments.
In this presentation we will focus on the static network, showing how by making measurements more rapidly (at 1 minute intervals), pollutants emitted locally can be distinguished from those due to longer range transport, leading to an innovative cloud-based method for remote calibration of the entire network for both gases and particulates.
In this presentation we will assess the performance of this method for PM, NO2 and NO against traditional approaches.
Scalable Spatio-Temporal Measurements and analysis of Air pollution data using Vehicle Mounted Sensors
Presented by: Charul Paliwal, IIIT Delhi
Summary: Measuring the local air quality is a reliable proxy of measuring the emissions in a given location. The measurement of air quality parameters in a locality is the first crucial step towards the localization of credible pollution sources like local industrial units, transportation, or even crop burning. Dense air quality monitoring in most cities/towns, especially in the developing countries, is virtually non-existent due to the expensive static sensors setup.
The central theme of this work is on answering the following questions: 1) How can we develop a dense spatiotemporal air quality map in a city using only few monitors? 2) Does building a network of mobile monitors along with static monitors help in creating a dense air quality map?
We propose the creation of a dense air quality map by performing a spatiotemporal sampling using only a few moving sensors followed by the suggested matrix completion technique. The underlying low rank and slowly time-varying structure of the air quality data can be leveraged to create models that facilitate an effective spatiotemporal extrapolation. These mobile monitors are put in the public transit buses as well as small vehicles to ensure dense coverge. We claim that to obtain a dense air quality map; the cities may require only a small fraction of moving sensors as compared to all static sensors setup. Therefore moving air quality measurement could lead to an effective strategy against air pollution and climate change.
We are further left with research questions on : 1) What is the best moving sensor strategy? 2) Should we put a mixture of accurate (industry grade) sensors and low-cost monitors? 3) How to calibrate moving sensors using the industry-grade static sensors 4) What cost savings can be delivered with such a setup? 5) Additionally, how do we localize the cause of pollution using such a setup?