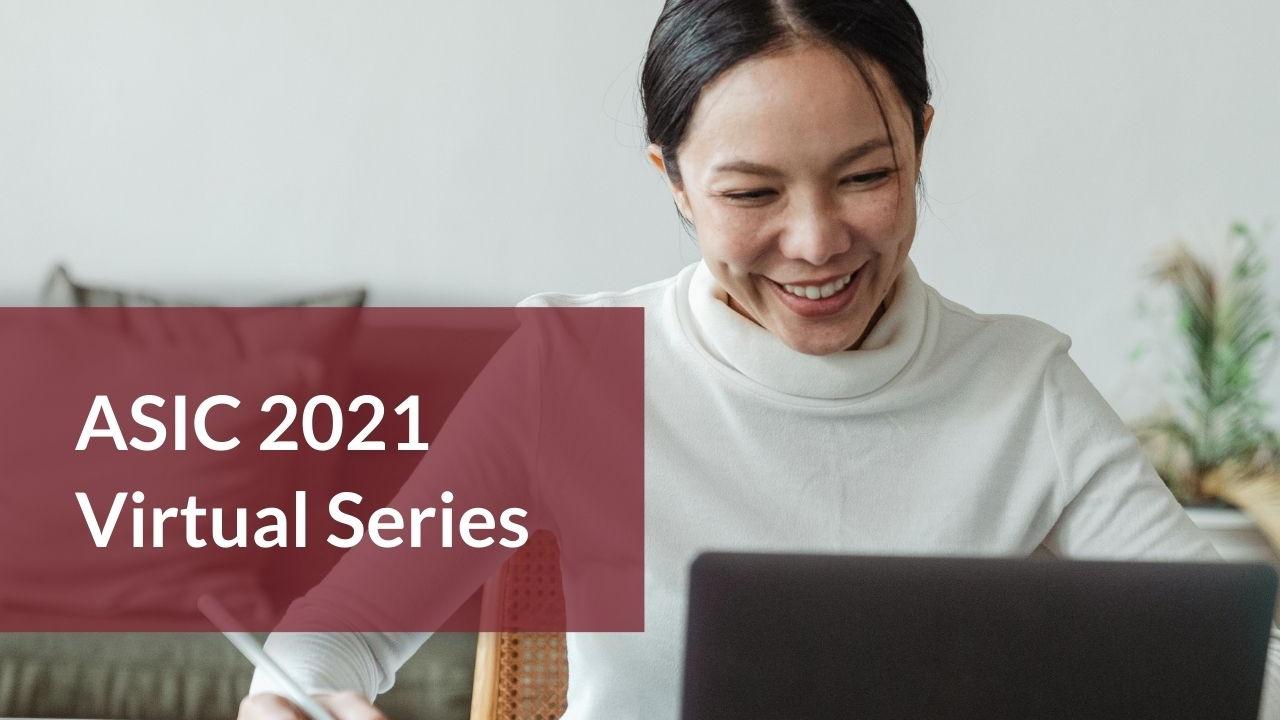
Event Date
Time: 8:00 a.m. PT / 11:00 a.m. ET - 9:15 a.m. PT / 12:15 p.m. ET
Description: For decades, air quality has been determined and communicated using data from high quality reference networks operated by government agencies and trained staff. Increasingly air sensors, operated by a variety of individuals, are offering estimates of air quality at neighborhood scales, in rural communities, and in countries where high quality reference networks may not exist. This data has the power to fill in gaps in our understanding of air quality but often, direct comparison of sensor and reference data is complicated because sensor data needs to be cleaned and corrected in order to provide data comparable to reference networks. This session will discuss the process of combining sensor and reference network data through the lens of three organizations approaching the integration of data in different ways with different goals and audiences in mind. This session will conclude with a panel discussion during which presenters will reflect on audience questions, their experience in using these tools to communicate air quality, and the future of these and similar efforts.
Moderated By: Andrea Clements, US EPA
Presenters
- Scott Epstein, SCAQMD
- Sim Larkin, USFS
- Karoline Johnson Barkjohn, US EPA
- Glory Dolphin Hammes, IQAir
- Yann Boquillod, IQAir
Presentation Abstracts
AQI Mapping Using Model, Regulatory Monitor, and Sensor Data in Real-Time
The public often uses multiple sources of air quality data to determine air quality conditions in their area. Proper interpretation of this data is especially important within the South Coast Air Quality Management District (South Coast AQMD), a region with 17 million people that typically records the worst air quality in the United States. With the widespread use of low-cost sensors within our jurisdiction, sensor data is commonly misinterpreted, as the accuracy, siting, pollutants measured, and any necessary calibrations are not typically considered by the public. To assist the public in the interpretation of various air quality data sources and characterize air quality levels throughout our jurisdiction, we developed a real-time air quality index map that blends model data, regulatory monitoring data, and calibrated/quality controlled low-cost sensor data. Blending is performed by weighting each data source by their relative uncertainty, providing air quality index (AQI) values at 5 km resolution that are considerably more accurate than previous methods, especially during wildfires. The real-time AQI map along with plain-language recommendations on how to minimize exposure during periods of poor air quality is currently available on the South Coast AQMD website (www.aqmd.gov/aqimap) and in the South Coast AQMD mobile app (www.aqmd.gov/mobileapp). PDF Slides
Follow Up Questions
- What are the statistical checks you apply to the two PurpleAir channels?
- Compare the differences between the A and B channels over a one week moving window using several statistical techniques to identify sensors with large bias or scatter between the two sensors. Details are provided in our paper in Environmental Research Letters (https://iopscience.iop.org/article/10.1088/1748-9326/abb62b/meta).
- This looks a bit like the methods used by some low cost sensor algorithms to correct their measured data. Would this corrected data be used by your algorithm and might the result be twice corrected?
- Our correction is applied to the purple air data before it is corrected. However, as we add more sensor types that have onboard calibration, we may consider including them directly.
- When you use LOO CV, do you specifically choose to leave out high-quality monitor data to compare with the low-cost data, or do you not discriminate between the two during CV?
- We only leave out high quality monitor data when doing LOOCV.
- How often is the AQ model every day to run to help integrate hourly PurpleAir PM2.5 data?
- Model is run twice daily by NOAA.
- Is this done in near-real time?
- Yes, hourly updates to the map.
- When combining monitoring data, are weather conditions considered such as wind speed and direction?
- We don’t consider wind speed and direction directly when doing the interpolation, but the model data that helps us interpolate O3 and PM2.5 takes this into account.
- For your integration of low-cost sensors using their uncertainty, what uncertainties are you using? Is it the same uncertainty for each sensor/location, or is it location-specific?
- This is location specific because the uncertainty is based in part on the variation of the PA measurements in a grid cell.
- Do you use some geographic boundaries for interpolation given complex terrain? (Evan Shipp)
- The AQ model assisting in the interpolation considers terrain.
- Is your map updated in near-real time?
- Yes, updated hourly.
- Does your agency itself deploy Purple Air Sensors and if so, do you have siting criteria?
- We do, and we are currently doing this to fill holes in the map. Our AQ-SPEC website has siting criteria on their website. See http://www.aqmd.gov/aq-spec/resources/related-documents for details.
- how the uncertainties were estimated? Which metrics are you using?
- Details are provided in our paper in Environmental Research Letters (https://iopscience.iop.org/article/10.1088/1748-9326/abb62b/meta).
- Does your system every have to go back and recalculate low cost data such that it changes from when it was initially disseminated. And if so, do you ever get questions from the public as to why data has changed?
- Since the low-cost sensor data only affects the map for a few hours in the Nowcast AQI calculation, any subsequent changes to the data would not affect the map unless it occured in that time window. We do not modify the low cost sensor data after it is collected.
- can you talk a little bit about how Ozone was considered in making the gridded system. Does the low monitor network include Ozone monitoring or just PM?
- Currently only PM2.5 low-cost sensors are fed into the map. Ozone AQI values are generated with our comprehensive regulatory monitoring network and a chemical transport model. We are in the process of deploying AQy sensors in collaboration with our AQ-SPEC group that will provide additional ozone measurements.
- Have you been able to compare with satellite data?
- We have not compared out data with satellite data. Since the ground measurements tend to be more accurate, a comparison may not be the best assessment of our accuracy.
- Have you considered generating maps of estimated uncertainty
- We haven’t considered this, but it is an interesting idea.
The AirNow Fire and Smoke Map Pilot Project
Sim Larkin, USFS & Karoline Johnson Barkjohn, US EPA
In 2020 the Environmental Protection Agency (EPA) and U.S. Forest Service (USFS) jointly developed a pilot project that significantly revamped the Fire and Smoke Map available through the EPA’s AirNow website. This newly improved map offers many enhanced features and differs substantially from other information available through AirNow in that it integrates and displays data from low-cost air quality sensors. The sensor data (from PurpleAir) is adjusted based on a correction equation developed from a dataset of PurpleAir sensors located along side air monitors across the country during both ambient and smoke-impacted conditions and additional quality assurance steps are applied. Overall, the Fire and Smoke Map saw significant usage during the historic 2020 smoke season with over 7.4 million page views within the first 3 months of the pilot effort. This pilot effort brought to light a number of challenges with incorporating multiple data sources such as data time resolution, uncertainties, and differences in the timeliness of reporting. USFS and EPA plan to improve upon lessons learned in 2020 as this pilot continues. NOTE: Although this abstract was reviewed by EPA and approved for publication, it may not necessarily reflect official Agency policy. Mention of trade names or commercial products does not constitute endorsement or recommendation for use. PDF Slides
Follow Up Questions
- What is the data interpolation scheme in this case?
- At this time, the Fire and Smoke Map avoids data interpolation and presents only data at the locations that the observations occur. Future development may include data interpolation but a schema has not been decided upon at this point. For the in your area display the closest 3 monitors and closest 3 sensors within 30 mi of the location are presented.
- How effective would networks of low-cost autonomous sensors be in detecting the start of wildfires if strategically placed (with batteries, solar PV and networked communication in real-time)?
- There are a variety of automated tools in current use and in testing for fire start detection but they are not air quality based. These range from interpretation of automated lightning strike data to automated visible cameras looking for smoke plumes rising vertically to infrared detections by satellites, aircraft, and UAVs. Detecting fires by inference from air quality typically requires a more developed plume
- How can we submit our EBAM data so it shows as a temp monitor on the map.
- 2 answers here. (1) Currently, any data identified as “public” in the AirNowTech system should get to us and be shown on the map. (2) For the future, we are working on an additional data submission pathway / standard designed to be simple to implement (develop a CSV or JSON type file and send it or let us grab it from your website), and hope to have this rolled out later this year.
- When are temporary E-BAM monitors set up? before the forest fire season or on a case-by-case basis?
- This depends on the policies of the agencies setting them up, but typically this is done on a case-by-case basis. For some planned burns, monitors are set up in advance. For wildfires, monitors will often be set up both in areas already experiencing air quality impacts, but also in nearby areas that are anticipated to be impacted.
- Where can I find more information on the US wide correction equation for PM10 rather than PM2.5? Was this used in this study?
- We have not built a correction equation for PM10. There are a number of recent papers from other groups suggesting that PurpleAir and Plantower PMS5003 don’t do a great job measuring the larger particles. This is likely due to the lower light scatter to mass ratio for larger particles and potentially challenges in sampling the larger particles by the sensor fans.
- Have you considered a "rolling" NowCast calculation, maybe updated every 5 min?
- We are currently working on a variety of different options to include sub-hourly data on the map. This is indeed one of the ideas that we are examining. However the NowCast averaging time does limit the strength of the low cost sensors in their ability to respond rapidly to changing conditions, so other ideas are being examined and discussed as well. If you have specific thoughts on this please send them in to epasenor
- Are sensor data being used in any EPA forecasting projects?
- I cannot answer this question as I’m not aware of EPA forecasting work.
- Does the correction factor have to be adjusted depending on changing MET conditions or is it a one edit fits all?
- It is one equation that includes a relative humidity term.
- The correction was applied to only fire days. What about the bias correction for non-fire days?
- The original correction was actually built based on 24-hr averaged PM2.5 data from across the U.S. with most data from typical ambient conditions (not impacted by smoke). We then tested on smoke impacted data and it works well up to ~250 ug/m3. We are in the process of building a non linear correction that will work for both typical ambient data, smoke impacts, and extreme smoke impacts.
- The A B channel exclusion causes sensors to be excluded even when one channel is well correlated with nearby sensors. Have you considered doing some spatial comparisons to include in your QA evaluations? (Evan Shipp-paraphrase)
- This is not a QA check we are using at this time. PM2.5 may be more or less heterogeneous during smoke impacted times and under different geographies and meteorological conditions. More work would be needed to ensure we did not bias our dataset by using spatial QA evaluations.
- Is there any way to retrieve the hourly values from the corrected sensor data being fed into the AirNow Fire & Smoke Map for our own analyses?
- For the permanent and temporary monitor data we have ways to do this both through web interfaces and through an R package. For the low cost sensor data we are not currently distributing these as they come from Purple Air. However this is a request we have heard and will try to address in some fashion after the deployment of the revised map this summer. At the least we should have codebase that will be shared that can be applied to data retrieved from Purple Air’s own data archives.
- How do you take into account the impact of the sudden and random baseline step changes in low cost sensors which have been identified by the EPA (and others)?
- We are able to remove sudden baseline shifts since we are comparing measurements from the duplicate Plantower sensors (chanels A and B) in the sensor. This is more challenging for sensors without duplicate measures as it can be unclear whether this is a change in concentration or a sensor malfunction.
- The sub-hourly is not linked to direct health studies, right?
- This is probably a better question for a health scientist but there is more research associating 24-hr and longer exposures to PM2.5 with health effects.
- The dissemination of all the AQ data seems also a great opportunity to educate the public about the PBL dynamics and its effects on local AQ. Is there anything planned along those lines?
- Agree. We haven’t developed material for the public on this topic (we have some for trained specialists), but if anyone has good resources or would like to partner in this development we would look for ways to link that into the map.
- Is there any way to look at historical data (past year/past few days) in either IQAir or AirNow?
- For the Fire and Smoke Map, not currently, although this is something on our list of desired functions for the future.
- As a California resident, I am dependent upon the fire map. Thank you for participating in developing an important tool. Climate change has created a new norm for California: megafires. Dangerous to us as discovered in the past few years.
- Glad you are using it and I hope you find it useful. Please contact sensordatapilot@epa.gov if you have any suggestions.
- What is your opinion on the PM measurement range for which the correction factors are calculated? Some correction factors (i.e., Wisconsin DNR) do not include meteorological parameters (such as: Temperature and RH), what is your opinion on this?
- It is important to build and evaluate sensor corrections over the full range of conditions you expect to use the correction over. The EPA U.S.-wide correction factor we developed seems to work well in the states we tested it in (https://amt.copernicus.org/preprints/amt-2020-413/). However, local corrections are likely better suited as long as they are built on a robust dataset. Ideally a local correction would be built based on at least 1 year of data across a few sites to capture the full range of PM2.5 and environmental conditions that it is expected to be applied over.
- Relative humidity will impact the light scattering per mass of particles differently depending on particle properties potentially making it more or less important in different parts of the country. It is less clear how temperature is related to sensor performance (although it may be related to sensor optical properties) and it can also be hard to include in a correction equation since it is typically strongly correlated with relative humidity (RH).
- Do the inexpensive PurpleAir sensors really produce data that compares well to more conventional sensors?
- Sim Larkin, USFS: A hard question to answer succinctly. In my opinion, they produce data that is useful, particularly where no other observational data exist, and their numbers and spatial distribution (both current and potential) make them a unique data source. In this way the value of the low cost sensor data as compared with its absence is substantial. Without them, various locations would require some sort of pure imputation -- permanent observational network, satellite, or model based, and the low cost sensors are better than what you get in that case; their ability to spatially locate the extent of impacts is substantial and unique, particularly when corroborated by other sensors nearby.
- Do any of the panelists know of any community-based project that has built their own low-cost AQ monitors?
- Sim Larkin, USFS: Not specifically, although there are regional deployments of various sensor types.
- With so many choices, as a local air quality specialist with both an ozone and PM FRM/FEM monitors, which app/website should we direct our locals during a fire smoke event? our website aspenairquality.com, fire.airnow, Iqair, purple air, etc?
- Sim Larkin, USFS: Each provides somewhat different displays and information, making this question hard to answer in the generic. I am happy to discuss the Fire and Smoke Map with you to see how it might fit your needs, and I suspect other panelists would be happy to do the same for their products. The AirNow Fire and Smoke Map is intended to meet this need during fire smoke events, and if there are things that would help it do so we are happy to get that feedback.
- What is the price range for what you consider low cost and high cost?
- Sim Larkin, USFS: I don’t have a specific price target but the common usage appears to be “a few hundred dollars or less” for low-cost sensors. Perhaps the real distinction in our discussions today relates to the number of sensors able to be deployed, which is directly related to whether the sensors are affordable and installable by a large number of households or primarily only by agencies.
- What is the tradeoff between doubting a high reading on a low cost sensor that might be a real local spike such as illegal burning, and suspecting that it might be an anomaly and suppressing or flagging iit?
- Sim Larkin, USFS: Speaking for myself, I believe that these types of questions are really about representativeness. A spike due to a source that might affect a broader area and persists (a large structure fire for example) is useful to capture and display; one that is due to a source that only affects a narrow area (a barbeque near the sensor) is not. Unfortunately the difference between these can be difficult to assess. Displaying this data but flagging it as potentially not being representative is a way to try to address this issue.
- Can any of the panelists talk about public confusion related to interpreting AQI, especially with different time scales?
- Sim Larkin, USFS: Several good comments were made by various panelists in the discussion section of the webinar and I would encourage anyone interested to reference that recording. For myself, I have dealt with confusion about what time averaging scales observational data represents and how that is best interpreted. However, this may be better understood less as confusion and more as an inherent tension in the timescales that the person is operating on vs. the timescales of the instrumentation and metrics shown. People often want to see monitored data reflect their immediate current experience. This is particularly true in rapidly changing situations, but can be true just due to the variable nature of smoke. Many of the decisions that people want to make are relatively short in duration: Can I go for my 30-min jog? When is the best time of day to get to the grocery store? However health effects have been developed over longer statistical averaging times, with reference levels developed from those health metrics. This creates the tension, and it is one that is still being analyzed, discussed, and worked on. It is also confounded by the variability in short duration data where moment to moment values can jump around substantially due to transient effects such as a bus passing by, etc…
- How are panelist might imagine these data sets being collected by local air sensors as a way to teach and inform our K-12 students through coursework in maths, sciences, and even social science?
- USEPA ORD has been working on several hands-on activity based lesson plans using air sensors to explore air quality. Currently 3 lesson plans are near release focusing on 1) outdoor air quality, 2) indoor air quality, and 3) personal exposure. Two additional lessons will be developed this year. These plans will be housed, along with other existing resources, here: https://www.epa.gov/air-sensor-toolbox/educational-resources-related-air-sensor-technology.
- Sim Larkin, USFS: This is a great suggestion. See above for some lessons from the EPA. While I don’t have a specific course in mind and am not sure what will be included in the EPA lessons, I would love to develop a more interactive exercise, perhaps using something like Jupyter Notebooks and code the kids could run and adjust themselves using real data. If anyone would like to work on this, my group would love to partner with you on it. We have large data archives and various codebases that we would be happy to share.
- Are emissions data necessary for accurate forecasting in cities? Or are historical data and meteorology enough?
- Sim Larkin, USFS: In my experience, the answer here depends on what situation you are discussing. First, it depends a lot on whether you are discussing PM2.5 or Ozone. Ozone tends to be more emissions sensitive. Additionally, it depends on if you mean “for a typical day” or “during a large event, like a wildfire.” For many places a reasonably good forecast for a typical day can be generated from historical data and a statistical association that includes day of the week, time of year, etc… During large events, such as a wildfire, however, emissions data is very valuable in setting the potential level of impact. This is true even compared with other fire related information such as fire occurrence or size data (fire emissions data generally outperform both of these).
- How do you see the relationship between real-time indoor and outdoor air quality measurement in the new building world?
- Sim Larkin, USFS: I suspect this is highly dependent on the specific building codes of the location. For example, in some areas building codes enforce or encourage the inclusion of more outside air into the building’s ventilation system to improve indoor air quality and for energy reasons. If these systems, once installed, are not capable of being shut off or the air intakes cannot be rerouted to include less outside air when high levels of smoke are present outside, this can create significant issues for indoor air quality. Additionally more temperate climates often have fewer air filtrations systems due to lessened need for heating / air conditioning, again making indoor air quality harder to maintain during smoke episodes.
Combining satellite data, low-cost and reference sensors using AI
Glory Dolphin Hammes, IQAir & Yann Boquillod, IQAir
IQAir has implemented a unique approach, powered by AI, to compute air quality data from combining satellite imagery, weather data, low-cost and reference monitors. IQAir visualizes this air quality data in simple to understand ways that engage individuals, communities and governments around the world. The IQAir data platform incorporates one of the largest number of low-cost sensor data points, which integrates this data in the highly-rated AirVisual mobile app. With the large increase of low-cost sensor data points, it was challenging to compute reliable and accurate air quality data. The presentation will cover how IQAir developed the AI models for a diverse set of data sources, as well as integrate them into a visually appealing map. PDF Slides
Follow Up Questions
- What type of machine learning algorithms are you using? How much prediction skill is provided using satellite AOD?
- Machine learning is used for 3 things: data validation + data calibration + forecast
- How localized is the IQAir forecast? 1 square mile? 1 city block? Larger or smaller?
- It depends on the location: 10x10km in low population area, down to 1x1km in certain cities
- Depending on the density of the local sensor network, the IQAir forecast shows the nearest air quality station or city (a cluster of stations).
- What is meant by gov't sensors need to be validated?
- While the US EPA sensors are mostly validated, it’s not the case of the government monitors in other countries.
- We validate (publish or do not publish) gov’t sensors, but do not calibrate (adjust based on parameters) gov’t sensors.
- Would one of the ways to get around the issue of calibration of low cost sensors to quote relative values for example that the values is twice the average for the last month. This may also be better understood by the general public.
- In order to provide accurate and reliable air quality, low-cost sensor data needs to be cleaned (calibrated). There is not an issue to do this with machine learning. IQAir platform processes millions of data points hourly.
- Here in Norwood OH we are using airbeam 2 for mobile routes in specific commuter time slots. Thank you for confirming the need for testing on the ground. Can any other low cost sensor be used with the airbag? How does a community get on board with this ground level rollout? Our Norwood Health Department is our fiscal sponsor.
- You can speak to one of our Air Quality Specialists and get set-up: AirVisual Enterprise Dashboard: Get In Touch | IQAir
- Do you have any plans to use of mobile low cost AQ sensor data? I was thinking of Olympic Marathons.
- We have similar projects in development at the moment.
- Do you use or plan to apply any framework to categorize Processing Levels (milivolts, raw concentration, corrected onboard, corrected on cloud, etc) of the data you ingest from different vendors?
- We isolate this data on the frontend and backend, so that users can access their raw and corrected data.
- Does Iqair use purple air, monitors or other sensors for to inform their app?
- We use PurpleAir, IQAir, Clarity, and Beam Attenuation Meter (BAM). We strive to incorporate many types of sensors/monitors in order to provide data to as many global users as possible.
- You discussed a more global approach and I was wondering if different types of low cost sensors also differ in reliability and how you account for so much complexity. Also, do you encounter any challenge in gathering data from other countries?
- Using different AQIs in other countries is challenging, therefore we use the US AQI globally to comparisons easy. Some sensors are more reliable than than other. We currently only incorporate three types of low-cost sensors because there are differences in reliablity.
- Could you please tell us more about your Clean Air for kids program? Do you help improve indoor air quality for impacted communities such as environmental justice communities in urban areas?
- We help with Supplemental Environmental Projects (SEPs) and other programs with mitigation, providing clean air in schools and monitoring and environmentally impacted residential communities.
- For transparency and reproducibility, if we would use your data for research are your algorithms available
- Yes, we make this available for researchers.
- is your map showing NowCast AQI values?
- No, NowCast AQI values are different from the data on our map. We use machine learning to calibrate low-cost sensors, we update every hour with US AQI average.
- Why are Purple Air sensors the low cost sensors of choice given their challenges with longevity in dusty environments and humidity challenges? Given that there are now other options on the market that may have better performance?
- PurpleAir sensors provide high-level accuracy. However, the challenge with low-cost sensors are that they incorporate light-scattering technology, which is affected by humidity and pollution composition. SCAQMD has AQ-SPEC program that evaluates low-cost sensors: Evaluations (aqmd.gov)
- The scope is mainly external or also indoors? In the case of indoor environments, would the configuration and operation principles be the same?
- IQAir AirVisual incorporates outdoor and indoor air quality monitors on our platform for our users. However, we only make outdoor air quality sensors/monitors publically available. Indoor data is only available to that sensor user who owns that sensor or if that user shares it’s with a private share-code.
- How can local AQI readings be used to differentiate the health impact of industrial pollution versus pollen or smoke? What other information could be used to help provide community information?
- AQI readings are a great start to begin the air quality community conversation. That conversation can be elevated for industrial pollution to include PM 1 and black carbon, which is not often readily available. PM 1 and black carbon can provide meaningful readings that can affect health more than other air quality readings.
- Are you getting local government pushback on reporting poor air quality in their regions?
- We sometimes get pushback on reporting poor air quality data. It can be an embarrassment for local governments to report poor air quality. However, in terms of productivity, there is a saying: What gets measured, gets done. Therefore, it’s important that everyone can have free access to global air quality data to affect change.
- Is there any way to look at historical data (past year/past few days) from IQAir?
- Yes, we make this available for research. Please contact us Media Inquiries | IQAir.
Group Discussion